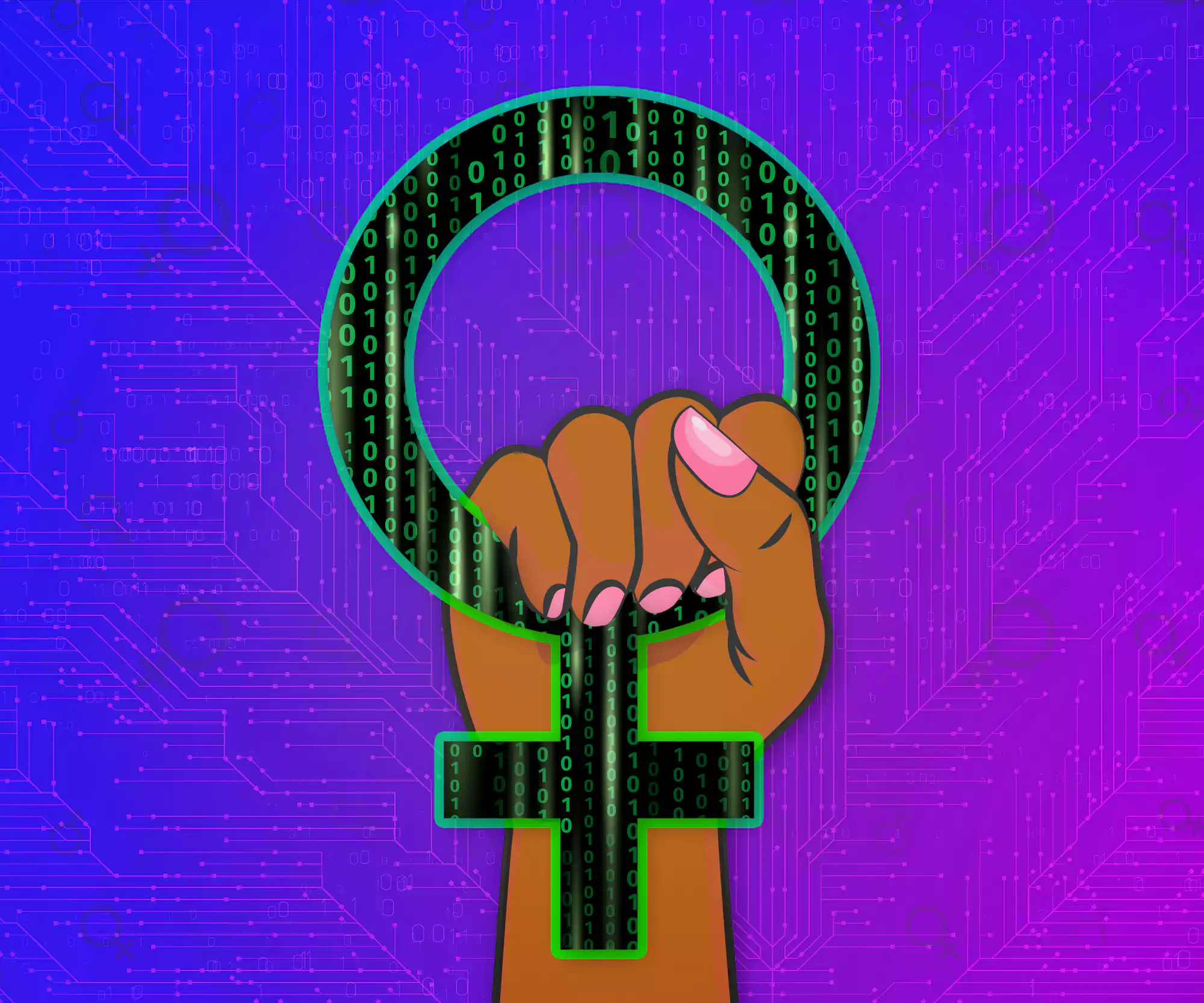
In a world where information floats around like an easy-to-access infinity pool, we often wonder—which source do we trust? Which finding is factual? On what premise is it all based? These serious questions lead us back to the crumbs lining our way: data. The exception is that the data we have access to are not crumbs anymore. Instead, they’re stockpiles of data gathered from far and wide through the latest and most innovative means possible. Qualitative data, quantitative data, big data, machine data—each an aisle of its own within a hypermarket of data. And as per market rules, the fast-moving data sits front and centre, packaged all shiny for its consumers. The hierarchy and bias are hard to miss. And that’s a problem—a problem that data feminism refuses to sweep under the rug.
What is data feminism?
Data science is the basis of essential policymaking decisions, among many other areas. For example, when establishing a welfare scheme, the government would need a social registry with real-time and historical data to determine what benefit will go to whom. Considering the far-reaching impacts of data science leads to some pertinent questions: who creates these data collections? Whose interests are kept in mind while doing so? What objectives lead the data collection? Are these objectives unprejudiced?
These are the questions that data feminism dares to ask.
The span of data feminism
Data feminism begins with the process of data collection itself. In a world where data yields power, it also becomes an essential tool for structural change. Collecting this data is bound to be driven by various agendas. In addition, biases in its analysis and presentation hinder social change or promote oppression. Joni Seager, a feminist geographer, has rightly asserted—“what gets counted, counts”. Collected data often becomes the basis for policymaking and resource allocation. Therefore, it is essential to look at the collected data from every angle and ensure that it is all-inclusive.
Feminism understands the need for inclusivity better than most. When we look at feminism in its most advanced form, i.e. intersectional feminism, it is not only just a belief but an action towards the equality of the genders. Let’s back up and understand intersectionality first. A term coined by Kimberlé Crenshaw, intersectional feminism is the idea that identities within and across social groups overlap and interplay, revealing that discrimination occurs at varying levels. So, a person can experience multiple degrees of discrimination on the one hand and privileges on the other. As such, when applied to data science, the feminist approach now seeks to challenge all systems of unequal power. Here are the ways in which data feminism attempts to overcome various challenges:
Ending asymmetrical power play
Data requires funding. So, unless the data is helpful to those who are putting in the money, i.e. corporates, universities, and government bodies, it doesn’t get collected or processed. Instead, a calculation called power analysis is usually conducted at the beginning of data collection to understand the sample size needed for the study. It considers a few key factors, such as the potential strength of the evidence gathered, whether it will have an effect, and how impactful that effect could be.
This analysis at every stage of the data creation process is necessary to truly understand the subjective nature of data. Only then can we know how all the decisions during the process brought about the final result. When this essentially context-based data goes into an AI algorithm, it limits how the data is made available to the end user. This limitation is ironic because a vital purpose of AI is accessibility to all. Since governments and organisations use data and data science for policymaking, asking the right questions can lead to better results and fewer blind spots. It’s important not to see these initial steps as burdensome because, ultimately, a biased algorithm is a bad one. Correcting the algorithm will produce better products and results and reduce the probability of error.
Freeing data from its binary confinement
It is possible to prevent errors in data using a classification system. By classifying data into binaries, the process of sifting through tons of it becomes easier. However, this classification fails to acknowledge that there may be exceptions (whether diversions or contradictions) to these binaries. The algorithm might then dismiss these exceptions as unreliable. Data is, therefore, not neutral.
We can see the subjective nature of data by analysing where, how, when, why, and who creates the data. Considering these different parameters often reveals that the process doesn’t consider non-binaries and margins. Data analysis draws fixed lines, and the grey areas must become black or white. We must break this rigidity for data collection and creation to make it more inclusive.
Data feminism’s foremost rule: equity > equality
In many parts of the United States, child welfare services use algorithms to determine which families are to be investigated for child abuse. However, in May 2022, Pennsylvania’s Allegheny Family Screening Tool (AFST) came under scrutiny. Experienced welfare officials found that the screening tool got it wrong one-third of the time. What’s more—it was racially biased.
Racism or sexism are not inherently part of these tools. However, its failure to include minority and underprivileged sections resulted in largely biased empirical evidence. Feminist data science recommends a more realistic approach instead. Rather than blindly ensuring the same access for everything to everyone, it makes more sense to know what needs are arising in which groups and cater to those needs specifically. This discerning method would have more immediate use than universal solutions which favour equality over equity. Viewing the current state of different groups in their historical context is imperative. If data confirms stereotypes, it’s important to ask why that is so and what other factors have led to these ‘stereotypical’ patterns.
Programming AI with race-blind or gender-blind data may yield racist and misogynistic results due to the subjective starting ground. For instance, it came to light that the first round of resume screening at Amazon eliminated those who had studied in women’s colleges. This elimination happened because the screening tool was meant to find people similar to those already working at Amazon. As you might imagine, because most of Amazon’s staff were white men, the algorithm was thus biased.
Feminist counter data
When the states fail to maintain and process essential data, feminists have taken it upon themselves to log in gender-based crimes. Most notably, María Salguero Bañuelos of Mexico has been logging femicides (gender-based killings of cis and trans women) because the government failed to keep any records. She spends 2-4 hours daily recording these deaths on a Google map which now has an extensive informant network. As a result, she has managed to compile the most comprehensive public archive of femicides, help families locate loved ones, and aid journalists and NGOs.
Activists are pressuring governments to count and measure gender-related crimes to form solutions. Many groups are doing this, especially in South America, not just for women but indigenous people. This kind of data cannot and must not be centralised because it is grounded in its context, which could get overlooked. Suppose we embrace a system that recognises plurality without attempting to take the ‘easier’ route of unifying data. In that case, the data will answer our questions more effectively because data analysis will not filter out the answers to form binaries.
Data feminism reveals pandemic realities
During the COVID-19 pandemic, it became clear that there was a lack of data about one specific group. We are, of course, referring to women in the divergent role of caregiver, hefting all the responsibilities of domestic chores and child-rearing—collectively called reproductive labour. Those who work within households, particularly as unpaid labour, are not visible in a capitalist system. In such a system, monetary worth determines the value of work. So, things that cost more are more valuable. But the value of invisible labour cannot be discarded—in fact, reproductive labour allows market production to continue.
The fact that there is no data about the large segment of society involved in this invisible labour is shocking. The Library of Missing Datasets by Mimi Onuoha (2016) features lists of essential data that haven’t been collected because they haven’t been given the attention they deserve. The list includes ‘People excluded from public housing due to criminal records’, ‘Trans people killed or injured in instances of hate crime’, ‘Muslim mosques/communities surveilled by the FBI/CIA’ and many more. As Onuoha explains, “The word “missing” is inherently normative. It implies both a lack and an ought: something that does not exist but should.”
Does data feminism determine the future?
Catherine D’Ignazio and Lauren F. Klein have put forth in their book Data Feminism seven principles of data feminism:

Considering these principles will elevate data science and eventually make visible those whom history and the world choose to forget. However, in light of intersectional feminism, there is also a need to look at the effects of data exposure. For instance, data about a minority group like transgender or queer people from a small community could expose them to violence. While representation is essential, data needs responsible and discreet handling.
Solution-building and creativity are essential to data science. As challenging as it may seem, those who deal with data must examine their methodologies. Feminist design for data tools or systems must consider those at the margins. They must primarily regard those who are the most affected. These principles will help data science become the tool we all hope and wish for positive change.